題目: |
Computational Intelligent Brain Computer Interaction and Its Applications on Driving Cognition |
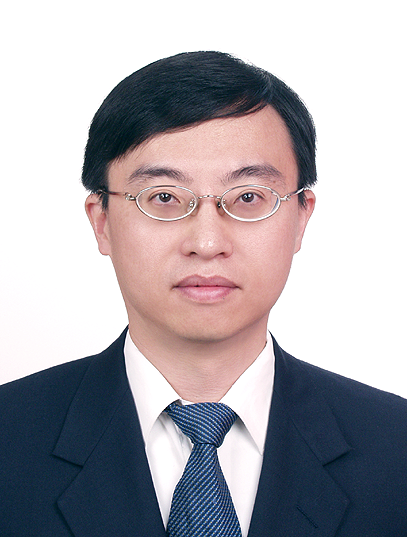 |
演講者: |
林進燈 講座教授 |
學經歷: |
國立交通大學電機與控制工程學系講座教授
美國普渡大學電機博士
國科會97年度傑出特約研究員獎
|
專長: |
Computer Vision, Fuzzy Neural Network, Speech Processing and Control, Brain-Computer Interface |
摘要: |
Human cognitive functions such as perception, attention, memory and
decision making are omnipresent in our daily life activities. For
instance, driving is one of the most common attention-demanding tasks
in our daily routine. Driver’s fatigue, drowsiness, distraction or
motion sickness is reported as the some of major causal factors in many
traffic accidents. When drivers lost their attention, they had
appreciably reduced (or diminished) the perception, recognition and
vehicle control abilities. Based on these causalities, how to
effectively prevent and enhance the human cognitive functions has
become a very important issue. Recently, many investigators had
developed novel algorithms based on computational intelligence (CI)
technologies such as Fuzzy Logic and Fuzzy Neural Systems to monitor,
maintain, or track the human operating performance. In this lecture, we
briefly introduce the fundamental physiological changes of the human
cognitive functions in driving first and then explain how to utilize
these main findings to develop the monitoring and feedback systems
based on Fuzzy logic and Fuzzy Neural technologies in the following two
topics: (1) EEG-based cognitive state monitoring and prediction by
using the self-constructing fuzzy neural systems; and (2) Spatial and
temporal physiological changes and estimation of motion sickness. These
research advancements can provide us new insights into the
understanding of complex cognitive functions and lead to novel
application enhancing our productivity and performance in face of
real-world complications. The achievements are a natural follow up to
the special issue on Brain-Computer Interactions, recently published in
the November 2009 issue of IEEE Computational Intelligence Magazine. |
題目:
| Recent Studies on Algorithms for Fuzzy Clustering |
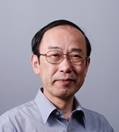 |
演講者: |
Sadaaki Miyamoto |
學經歷: |
Professor, University of Tsukuba, Japan
1978, Ph.D., Applied Mathematics, Kyoto University, Japan
2007, Fellow, International Fuzzy Systems Association (IFSA)
2009, Fellow, International Society of Management Engineering (ISME)
|
專長: |
Fuzzy Logic, Cluster Analysis, Data Mining |
摘要: |
Cluster analysis or data clustering has long been studied but recently
many more researchers are interested in this area of studies, as data
mining techniques are recognized to be very important in a variety of
sciences and engineering. In this talk we overview recent studies on
new methods and algorithms for fuzzy clustering, by focusing upon their
theoretical aspects. We first note that fuzzy clustering can be divided
into two categories of hierarchical fuzzy clustering and
nonhierarchical fuzzy clustering. The former theory was established in
1990, when an old method of the single linkage in agglomerative
hierarchical clustering is proved to be equivalent to the transitive
closure of a symmetric fuzzy relation. Moreover a key concept for the
equivalence is connected components of a fuzzy graph. After briefly
noting this, we move to the discussion of the best-known method of
fuzzy c-means clustering. We note there are two major objective
functions of fuzzy c-means clustering that use a basic alternative
optimization procedure with respect to cluster centers and membership
matrix. The well-known objective function has been proposed by Dunn and
Bezdek, while the other uses an additional term of entropy. The latter
has been proposed by a number of researchers. We show these two methods
have different theoretical properties by using fuzzy classifiers
naturally derived from the two membership matrices. Although the method
of entropy is less-known, we emphasize its importance in theoretical
and methodological sense. Next, two recent studies on clustering are
overviewed. First is clustering using kernel functions that are used in
support vector machines. How kernel functions are used in fuzzy c-means
clustering and related methods is described. Moreover cluster validity
functions with and without kernel functions are shown and a simulation
study comparing the effectiveness of different validity functions using
many numerical examples is mentioned. |
|